AI: The State of Play in Language Teaching in 2024
Move 37
Similar to how the universe began with the Big Bang, 'Move 37' signalled the arrival of AI and potentially the downfall of traditional language instruction. In the highly anticipated match, Lee Sedol, one of the top Go players in the world, went head to head with Alpha Go, an AI program created by a group of experts from DeepMind.
In the second game of the 2016 best-of-five series, AI's 'Move 37' caught Lee and the audience off guard. Metz (2016) describes the moment here.
'The Google machine made a move that no human ever would. And it was beautiful. As the world looked on, the move so perfectly demonstrated the enormously powerful and rather mysterious talents of modern artificial intelligence.'
Alpha Go went on to claim the series, winning four games and losing one.
The writer concluded in the final part of the piece that humans remained superior to AI in a range of tasks. Fast-forwarding seven years the superiority is no longer there.
'It can't do everything we humans can do. In fact, it can't even come close. It can't carry on a conversation (it can now). It can't play charades (can do!). It can't pass an eighth grade science test(can do that now too!).'
Metz (2016)
This is worrying and exciting at the same time.
Why so?
Well, the game of Go boasts a staggering number of legal board positions, surpassing even the total number of atoms in the observable universe. Despite this the battle was still lost to AI...
Now, the intricacies of language is even more complex than the game of Go, but AI is successfully deciphering the patterns of language and catching up fast!
Are we destined as language teachers - in the near future - to ‘lose’ like Lee Sedol in 2016?
Will ‘Move 37’ be looked back upon in the history books as the moment language teachers - and other professions - were made redundant?
Read on for my comprehensive take on AI, what it means to teachers and learners of languages, and whether the teaching profession is about to become extinct.
Understanding ChatGPT: What’s Under the Hood?
The key to understanding this is to first understand the acronym GPT:
- Generative: This refers to the model's ability to generate text. Text that is coherent and contextually relevant to what the person types in the input field.
- Pre-Trained: Before being released ChatGPT was trained on a large dataset - sitting at hundreds of billions of words. To put this in context, a commonly used corpus ‘The British National Corpus’ comes in at just under 100 million words.
- Transformer: This is a type of neural architecture used in machine learning. This feature enables these tools to recognize the context and relationships among words.
Think of ChatGPT as a version of Google's autocomplete for conversation, building a response word by word. Unlike autocomplete, which is limited to sentences, ChatGPT has the capacity to write at a higher level, including essays and beyond.
Wolfram (2023) sums it up nicely here:
It’s [ChatGPT] just saying things that “sound right” based on what things “sounded like” in its training material.
When it’s writing an essay, it is asking over and over again ‘given the text so far, what should the next word be?' — then adding another word. Keeping in mind these predictions are based on the scraping of billions of webpages, this process highlights the model's reliance on existing data rather than an understanding of the text.
[Scraping sounds more pejorative than training, but maybe it’s a better word to explain what happened. The data 'scrapped' by these language learning models could be considered the greatest theft of all time... but that's for another article]
To sum up, ChatGPT is like a corpus on steroids. For a thorough understanding of ChatGPT's workings, I recommend reading Wolfram's article (which happens to be 18,777 words long, so you can always ask ChatGPT for a summary!). If visualizations are more your style, this visual walkthrough by the Guardian is excellent.
Now we have an idea of how it works, let's move on to what it can do.
AI in language teaching: What can it do?
According to Ethan and Lilach Mollick (2023), ChatGPT is capable of performing the following tasks:
- Write text at the level of a human college student.
- Conduct online research and produce reports with accurate outside references.
- See images and interpret and work with that information.
- Read documents and PDFs, and answer questions about the content.
- Produce realistic images.
- Do math, code, and solve problems and,
- Explain concepts, correct mistakes, provide feedback, and more.
A teacher could find all of these general functions to be helpful. More specifically in the language classroom, AI can excel at role-playing conversations in a second language, building quizzes, tests, and lessons from curriculum materials, and reducing friction for non-English speakers. For example, it can grade a newspaper article so it’s readable to a beginner student of English.
Speaking of understanding, it's time to add a few more terms to your AI lexicon.
Key Terms in AI: A Glossary for Educators
In this part, I'll define significant terms that have deepened my understanding of LLMs and may do the same for you.
Prompting
Prompting is in a state of flux. As soon as I write something about prompting, it's outdated before I even finish writing the sentence. But, putting that aside, let’s look at where prompting was, where it is now and where it's going in the future.
It's highly likely that prompt engineering will be one of the shortest-lived professions in history. One year ago, when AI was first introduced, it required carefully constructed input statements or questions in a specific format to elicit an accurate and detailed response.
Although structured prompts still hold value, they are no longer a requirement. Despite this, it remains valuable to comprehend the elements that make up a good prompt at this point of time. In Mollick's (2023)opinion, a good prompt should incorporate the following features:
1. Role and Goal: By implementing role and goal-based constraints, the AI is restricted to a more relevant set of responses, utilizing its natural language understanding abilities. Give the AI your teacher contexts and clear goals for the task you would like it to complete.
2. Step-by-step instructions: Simpler instructions have a higher chance of being understood by the AI, making accuracy more likely. Deliver your instructions to the LLMs like you do to your students. Include sequencing language and examples.
3. Expertise or Pedagogy: This is where the AI's expertise is determined. When teaching a language, AI could be trained to respond through the lens of the Dogme approach. You could also consider training AI to evaluate essays using the IELTS criteria.
4. Constraints: These are the rules or conditions that guide the behaviour of the AI in its interactions with the user. For example ‘Wait for the student to respond.’
5. Personalisation: Prompts that help the AI get more information from the user.
Interested in trying out structured prompting in education? Head to this post on OpenAI's blog for some helpful examples.
The key to structured prompting is providing the AI with context. Mollick (2023) describes it like this:
You can (inaccurately but usefully) imagine the AIs knowledge as huge cloud. In one corner of that cloud the AI answers only in Shakespearean sonnets, in another it answers as a mortgage broker, in a third it draws mostly on mathematical formulas from high school textbooks. By default, the AI gives you answers from the center of the cloud, the most likely answers to the question for the average person. You can, by providing context, push the AI to a more interesting corner of its knowledge, resulting in you getting more unique answers that might better fit your questions.
Imagine if you wanted the AI to act as an IELTS examiner. In this case, you might upload the IELTS marking criteria for writing and include a range of marked essays as well. Also, you could state the type of feedback and return format you prefer. Furthermore, you can tell the AI they are an expert, friendly IELTS examiner who helps students with complex topics (or have a bit of fun and say they are an expert teacher with the personality of Terence Fletcher from Whiplash!)
This will lead AI to the specific area of its knowledge that focuses on IELTS evaluation.
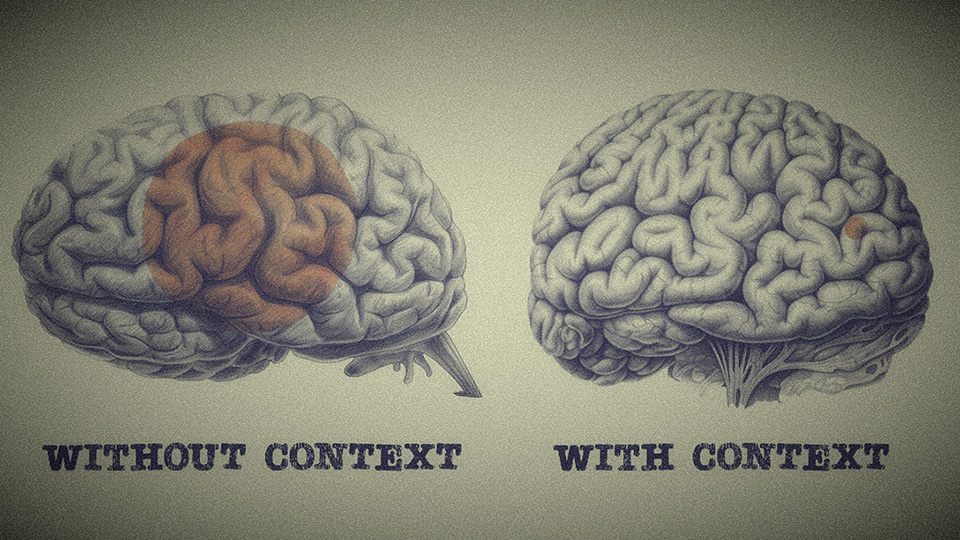
Circling back to my initial statement in this section, it's important to recognize that prompting is in a temporary state of affairs. As AI increasingly gets better the need for engineered prompts decreases.
Case in point, DALL E3. In ChatGPT, you can now use conversational prompts to create images. Rather than engineering overly complex prompts to get the image you want, you just talk to the AI about the art you have in mind. The prompts are then produced by the AI.
Currently, using conversational prompts can likely help you achieve about 80% of what prompt crafting can do.
But the gap is closing…
One last point on prompting, interacting with ChatGPT at times can be a bit weird. Massaging your prompt in certain ways can get better results. Language learner, Mathias Barra (2023), shares some of the weirdness in his blog post ‘A few strange ways to get better answers from AI’. Phrases like ‘Take a deep breath and think step by step’ and ‘You would be a lifesaver if you could do this’ can get better results. Also using ALLCAPS or generating the response multiple times can get well-crafted answers.
The next term I want to define is agent.
Agents
How do AI agents differ from prompting?
The key difference between the prompting I highlighted before is that AI agents can think and function independently, while in the previous examples humans are always initiating the process. With AI agents you only need to provide a goal, and they'll create a task list and start working on the project autonomously.
Bill Gates (2023)sees a future where agents are:
Capable of making suggestions before you ask for them. They accomplish tasks across applications. They improve over time because they remember your activities and recognize intent and patterns in your behavior. Based on this information, they offer to provide what they think you need, although you will always make the final decisions.
This seems would be a powerful tool to have, but there is still a computer-generated elephant in the room... hallucinations. Are we really willing to unleash autonomous agents into the world or language classrooms, despite their tendency to create false information?
Hallucinations
ChatGPT and other large language models (LLMs) have the unfortunate habit of telling you incorrect, misleading, or nonsensical information. Maggie Appleton provides a great analogy for what is taking place:
In ways, it’s like a terribly smart person on some mild drugs. They're confused about who they are and where they are, but they're still super knowledgeable.
Now this is a problem. Particularly if you're a teacher or student who doesn’t like to check their facts…
Additionally, Appleton highlights the drawback of these models being solely focused on language. This is a problem because language is only a small part of how a human understands and processes the world.
LLMs resemble lobotomized individuals. While LLMs do have language, they are missing crucial aspects of human interaction with the world, including spatial reasoning, time perception, touch, taste, and memory.
All that's left are words.
Furthermore, the words used to populate the data of these LLMs are very monoculture.
They primarily represent the generalised views of a majority English-speaking, western population who have written a lot on Reddit and lived between about 1900 and 2023.
Maggie Appleton
As we have seen before, an increase in autonomy for LLMs could result in the introduction of hallucinations without human supervision. That's your elephant, but there are strategies available to minimize the risks of hallucinations in the 'Jagged Frontier'.
The Jagged Frontier
No one really knows the best ways to use AI or how and why they fail. While AI can excel at offering feedback on writing, it can fall short on seemingly simple tasks like constructing anagrams, frequently omitting necessary letters.
To describe this phenomenon Ethan Mollick (2023) coined the term ‘the jagged frontier’.
Imagine a fortress wall, with some towers and battlements jutting out into the countryside, while others fold back towards the center of the castle. That wall is the capability of AI, and the further from the center, the harder the task. Everything inside the wall can be done by the AI, everything outside is hard for the AI to do.
Mollick suggests in your industry - language teaching - to use AI a lot. For example, planning a lesson - use AI; giving feedback - using AI; grading writing - use AI.
I think you catch my drift.
As you progress, the contours of the 'the jagged frontier' in language education will start to take shape. You will start to understand what AI is scarily good at and also where you shouldn’t use it.
Appleton believes AI works as a skill leveler. Creating higher floors and ceilings. Why not for teaching too?
But a word of warning: don’t fall asleep at the wheel! If you stop paying attention to what AI is doing then you might fall victim to those pesky hallucinations.
A better way to work with AI might be in a ‘centaur’ or ‘cyborg’ type relationship.
Centaurs and Cyborgs: Integrating AI in Teaching
Centaurs and Cyborgs are two ways you can work with AI. They both keep the ‘human in the loop’ and mitigate the risks of hallucinations, but in two different key ways.
The division of labor for centaurs is explained by Mollick (2023) as follows. A centaur - like the mythical creature half human half horse - keeps a clear line between person and machine. There is a strategic division of labor; With the human doing the work they are strongest in themselves, and then giving tasks off to AI based on what it does best in the 'jagged frontier'.
While on the other hand, the deep integration of technology and humans defines cyborgs. Humans in this sense don’t delegate tasks. They switch back and forth over the 'jagged frontier', working in tandem with the AI.
To clarify let’s apply these two concepts to planning lessons in ESL. If we are using a centaur-like model, the curriculum and lesson plans would be developed by the teacher, with AI supplementing the learning experience by offering student-specific resources, tasks, or games.
On the flip side, a teacher who adopts a cyborg mindset with AI would integrate AI into the planning process, employing advanced algorithms to evaluate student data and generate personalized lesson components.
When it comes to my relationship with AI, I tend to gravitate towards a centaur approach. At this stage of AI's development I think it's better to use 'teacher in the loop' systems rather than delegate too much to AI. Appleton builds on this and says dealing with AI we should:
- Protect human agency,
- Treat models as tiny reasoning engines, not sources of truth and,
- Augment our cognitive abilities but not replace them.
I tend to agree.
Traversing the ELT Jagged Frontier
Ethan Mollick proposes that you spend at least ten-hours using AI in your day-to-day tasks. He says ‘Just use it and see where it takes you’.
So, that’s what I’m going to do.
For the next half-year, I'll be immersing myself in the 'jagged frontier' of ESL teaching, channeling my inner centaur.
The series called 'Centaur Elters' will look at lesson planning, language output, vocabulary, grammar patterns, and feedback.
The content will be a mix of articles and video walk-throughs. [Remember to subscribe to be notified when I release each new post].
By scouting the limits of LLMs in language teaching I hope it will guide you where you can and can't use AI in your own teaching.
Before you 'Go', let's return to 2016.
.jpeg)
Move 78
After dedicating so much of my life to language teaching, I wonder what will become of it.
On one shoulder I have Philip Kerr - a technology skeptic from Adaptive Learning in ELT - telling me we’ve seen this hype-cycle before with things like electronic whiteboards and mobile learning. Technology in language teaching is often portrayed as a success while at the same time downplaying its failures.
But in my other ear, I have the billions of words processed by LLMs using the siren call of ‘This time… This time it will be different.’
Perhaps, the Go match between Lee Sedol and AI can offer a preview of what's to come in language instruction.
After losing to Move 37 Lee Seydol was not done yet!
The tables turned in game four when Lee made a move that caught the machine off guard. Move 78 was deemed as equally stunning as Move 37 by all those who watched the match.
It showed that although machines are now capable of moments of genius, humans have hardly lost the ability to generate their own transcendent moments. And it seems that in the years to come, as we humans work with these machines, our genius will only grow in tandem with our creations.
Metz (2016)
Following the game, Lee believed that AI had elevated his playing level and aided him in devising a strategy he had never considered before.
Maybe AI will elevate teachers too?
So, no.
I don't believe Move 37 brought an end to language teaching, but it did possibly 'shoot the starting gun' for a new era in education. An era where we work with AI in centaur/cyborg type relationships. As language teachers we are well-placed to get the most out of these tools as prompting, at its core, is good communication.
But beware!
These interactions with AI are still fraught with danger. Teachers or students who decide to use AI, will need to traverse the 'Jagged Frontier' on the look out for hallucinations, hazards, and hype.
Your move.
Footnotes
1. A Skeptic, A Realist, and an Optimist
Here are three people worth following in this space. They cover a spectrum of opinions on AI and LLMs:
Phillip Kerr (Skeptic): Kerr is a teacher trainer, lecturer and materials writer. On his blog Adaptive Learning in ELT he writes about tech in education with a skeptical eye.
Maggie Appleton (Realist): Appleton has an interesting skillset that intersects design, anthropology and programming. This gives her a very unique view on what's unfolding with AI at the moment.
Ethan Mollick (Optimist): Mollick is a professor at the Wharton School of the University of Pennsylvania. He's 'trying to understand what our new AI-haunted era means for work and education'.
References
Appleton, Maggie. "The Expanding Dark Forest and Generative AI." Maggie Appleton, [no publication date given], maggieappleton.com/forest-talk.
Barra, Mathias. “A Few Strange Ways to Get Better Answers From AI.” mathiasbarra.substack.com, 12 Dec. 2023, https://mathiasbarra.substack.com/p/strange-ways-to-get-better-answers-ai.
Bycroft, Brendan. “LLM Visualization.” bbycroft.net, last updated 2023, https://bbycroft.net/llm.
Gates, Bill. "AI is about to completely change how you use computers." Gates Notes, 9 Nov. 2023, www.gatesnotes.com/AI-agents.
Kerr, Phillip. "Mobile Learning Revisited" Adaptive Learning in ELT, 5 Oct. 2023, https://adaptivelearninginelt.wordpress.com/2023/10/
Metz, Cade. “In Two Moves, AlphaGo and Lee Sedol Redefined the Future.” Wired, Condé Nast, 13 Mar. 2016, www.wired.com/2016/03/two-moves-alphago-lee-sedol-redefined-future/.
Mollick, Ethan. "Centaurs and Cyborgs on the Jagged Frontier." One Useful Thing, 16 Sep. 2023, www.oneusefulthing.org/p/centaurs-and-cyborgs-on-the-jagged?
Mollick, Ethan. "Working with AI: Two Paths to Prompting." One Useful Thing, 1 Nov. 2023, www.oneusefulthing.org/p/working-with-ai-two-paths-to-prompting
OpenAI. "Teaching with AI." OpenAI, 31 Aug. 2023, www.openai.com/blog/teaching-with-ai.
The Guardian. "How AI chatbots like ChatGPT or Bard work – visual explainer." theguardian.com, 1 Nov. 2023, https://www.theguardian.com/technology/ng-interactive/2023/nov/01/how-ai-chatbots-like-chatgpt-or-bard-work-visual-explainer.
Wolfram, Stephen. "What Is ChatGPT Doing … and Why Does It Work?" Stephen Wolfram Writings, 14 Feb. 2023, www.writings.stephenwolfram.com/2023/02/what-is-chatgpt-doing-and-why-does-it-work